In Content Analysis, Coding is the key step and Categorical Thinking is the central tenet. The operational procedure is to transform textual data into quantitative data for subsequent statistical analysis. In many research practices, the combination of quantitative and qualitative data interpretation is often used to achieve the research purpose.
In traditional content analysis methods, coding involves manually reading the text, filling out coding sheets, and after completing all the coding, aggregating and organizing the data from the codebook for statistical analysis and visualization. This process is very time-consuming and labor-intensive, causing a lot of frustration for many users.
To address the cumbersome coding process in traditional content analysis methods, the R&D team of the DiVoMiner platform has come up with various solutions. Ultimately, they have decided to adopt an innovative approach that combines traditional content analysis methods with big data technology. In terms of coding, the platform provides two options: machine coding and machine-aided manual coding.
What are the differences between these two methods of coding? How should one make a choice? To understand this question, it is necessary to first grasp the differences in execution and the advantages and disadvantages of these two approaches.
The key factor in deciding which coding method to choose: is the attributes of codebook categories.
Machine coding is recommended for objective category questions, which is efficient and reliable;
For subjective category questions, machine-aided manual coding is recommended for high accuracy.
- When to choose machine encoding?
Objective topics such as institutions, personal names, events, etc., which have clear expression and can be summarized semantically, are highly suitable for machine coding. It is highly efficient and saves a lot of time and effort. With the reliability check of algorithmic robots, the coding results can be reliably used in academic papers and published in high-level journals.
- When to choose machine-aided manual coding?
If the codebook cateregories tend to be subjective, current technological capabilities make it difficult to make accurate judgments, such as subjective opinions of specific individuals or ambiguous attitudes. In these scenarios which cannot be quickly determined through machine coding, it is recommended to perform manual coding. By utilizing certain machine-aided tools, the efficiency of coding can be relatively improved.
Sometimes in practice, a codebook may contain both objective and subjective categories. In such cases, a mixed coding approach can be used to handle the data processing.
In fact, machine-aided manual coding, machine coding, and machine learning coding are all part of the big data technology-assisted content analysis method, suitable for different textual data research scenarios. Next, let’s view a detailed introduction to machine-aided manual coding and machine coding.
- machine-aided manual coding
As the name refers, manual coding involves human coders reading the text and filling out the codebook to complete the coding process. This method is suitable for analyzing subjective categories. Traditional content analysis methods operate in this way, requiring a significant amount of human resources and time, and relying heavily on manual effort. Therefore, it is not suitable for large-scale data analysis tasks that require high efficiency.
To assist users in completing manual coding more easily, the DiVoMiner development team has developed a “machine-aided” feature on top of traditional manual coding. Users can set keywords for category options (click for information about category settings). The set keywords will be highlighted in the coding text and machine suggestions will be provided, reducing the effort required by coders to search for and match information while reviewing the text. Even with manual coding, compared to traditional coding methods, there is a significant improvement in efficiency and accuracy.
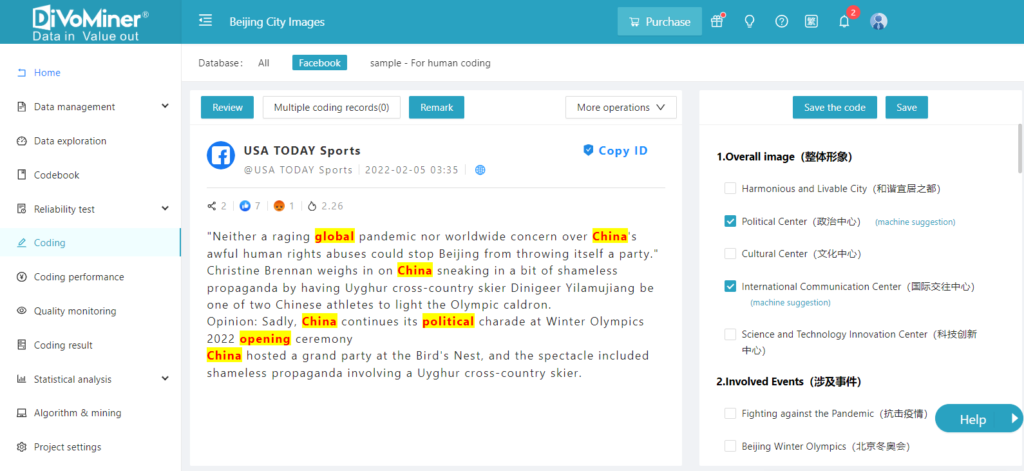
The accuracy of manual coding relies on the understanding of the coders. To ensure the reliability of the data, it is necessary to conduct intercoder reliability testing among coders before the formal coding process. For more information about intercoder reliability test, click to review it here.
- Machine coding
In big data research scenarios, the data samples used can often reach thousands, or even millions entries. Manual coding struggles to handle such large volumes of data and becomes challenging to sustain. Therefore, there is an urgent need for machine assistance in data analysis.
For objective categories in coding, where the semantic categories of the categoriy options can be summarized in text, it is recommended to use machine to perform textual classification tasks. This can be done with a single click, automatically filling out the codebook based on user-defined keyword logic conditions. This approach is fast, efficient, and capable of processing thousands of data entries in a short period of time. Furthermore, the results obtained through repeated executions are consistent and easy to verify.
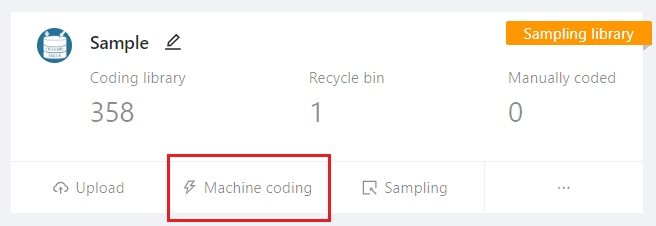
It is important to note that machine coding does not completely delegate all tasks to the machine. The results of machine coding highly depend on the user-defined keyword conditions, and “human” plays a significant role in this process. The crucial step where human intervention is required is in establishing the standard for machine identification. Therefore, the user’s research abilities, experience, and expertise can greatly influence the quality of the coding results. Machine coding allows researchers to free themselves from the tedious and mechanical works of manual coding, enabling them to focus their energy on research.
Note: Whether it is machine coding or the pre-selection in manual coding, the basis is determined by the user-defined keyword conditions within the category options. Therefore, the quality of the coding data still depends on the researcher’s research abilities and intellectual contributions. In a study, it may be necessary for the researcher to make repeated adjustments to the settings and correct the results in order to achieve a better coding outcome.